Where is the world rich in feedback?
As Ilya said at NeurIPS, we only have one internet. Once the fossil fuel of existing human-generated data has been consumed, further AI progress requires new sources of information. Broadly speaking, this can happen in two ways: search against a verifier, which trades compute for information, or through direct observation and interaction with the world. So if you want to predict medium-term AI progress, ask “Where is the world rich in feedback?”
There are two major dimensions for scaling AI: pre-training, and inference time scaling1. The most recent cycle of AI progress has been driven by inference time scaling (OpenAI O1). These models are trained with reinforcement learning (RL), which requires a reward signal. Reward is much easier to specify in some domains than others, which is why O1 shows huge performance gains in math and code (domains with verifiable right answers), but little to no improvement in more subjective areas such as writing.
Scaling pre-training with synthetic data is almost the same problem. For generated data to be a net win, you need some quality signal to filter it. So essentially all AI progress now turns on availability of scalable quality/reward signals2.
A skewed pattern of AI progress is therefore likely to persist in the medium term. The graph below shows some areas which have faster and cheaper sources of reward. Those seems like a good bet for where AI will move fastest3.
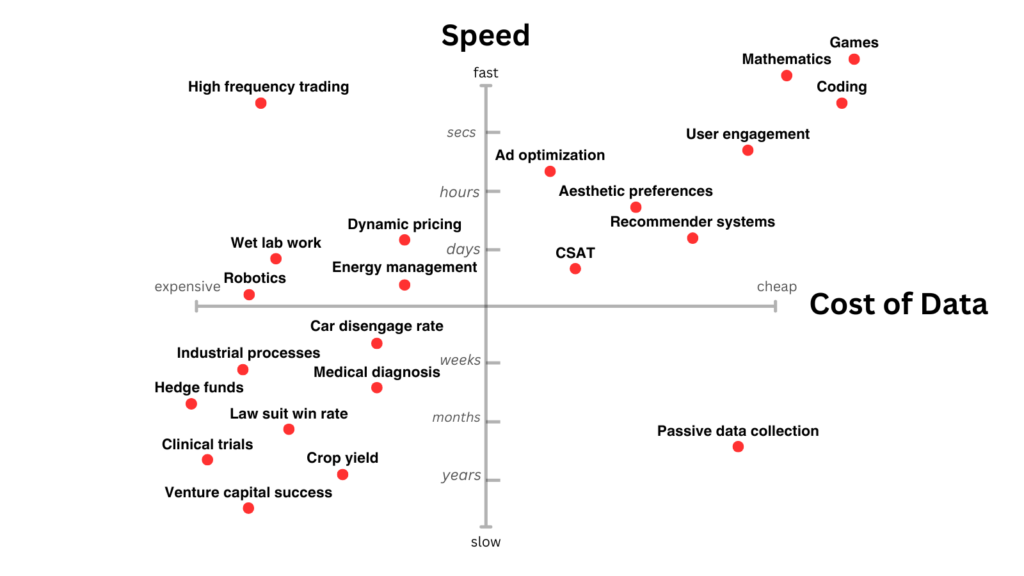
I’ll dig into a few of these areas, and then speculate about how some problems with less precise reward might be addressed.
More